Welcome to the webpage for Tweeki, an entity linking system for Twitter.
To identify what entities are being talked about in tweets, we need to automatically link named entities that appear in tweets to structured KBs like WikiData. Existing approaches often struggle with such short, noisy texts, or their complex design and reliance on supervision make them brittle, difficult to use and maintain, and lose significance over time. Further, there is a lack of a large, linked corpus of tweets to aid researchers, along with lack of gold dataset to evaluate the accuracy of entity linking. In this paper, we introduce (1) Tweeki, an unsupervised, modular entity linking system for Twitter, (2) TweekiData, a large, automatically-annotated corpus of Tweets linked to entities in WikiData, and (3) TweekiGold, a gold dataset for entity linking evaluation. Through comprehensive analysis, we show that Tweeki is comparable to the performance of recent state-of-the-art entity linkers models, the dataset is of high quality, and a use case of how the dataset can be used to improve downstream tasks in social media analysis (geolocation prediction).
Paper
We published the paper at the Workshop on Noisy User-generated Text (W-NUT).
@inproceedings{tweeki:wnut20, author = {Bahareh Haradizadeh and Sameer Singh}, title = { {Tweeki: Linking Named Entities on Twitter to a Knowledge Graph} }, booktitle = {Workshop on Noisy User-generated Text (W-NUT)}, year = {2020} }
Authors
Bahareh Harandizadeh
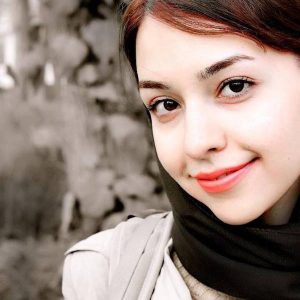
University of California, Irvine